A Review on the Use of Deep Convolutional Neural Network in Evaluating Oceanic Oil Spills
Oil spills remain a difficult calamity to rehabilitate due in part to the imperfect nature of the responses available. Even an optimal response following a spill leaves local ecosystems poisoned and local dependant economic activities diminished. Prevention is always preferred over recovery, as the immense direct, indirect and unknown damages can last decades. The responsible companies are largely held liable to costs associated with government agencies, public infrastructure repairs, lost income to other businesses, litigation and cleanup efforts. The average cost of the response is $16 a gallon. The 2010 Deepwater Horizon spill, the worst in US history, ran up to $476 a gallon ($60 billion in total) (Uhlmann & Liss, 2021). The damage to local environments, however, is difficult to gauge. In the wake of Hurricane Ida, the Coast Guard has received over 350 reports of oil spills along the U.S. Gulf of Mexico (Varghese, Arpan et al, 2021). Despite the decades-long trend of declining spill numbers and intensity, they remain a relevant issue. In order to limit damages, response organizers need to quickly and precisely identify affected areas in order to dictate the optimal course of action. Remote sensing specialists hope to enhance cleanup efforts through improved identification of oil-affected areas.
Synthetic Aperture Radar
Synthetic Aperture Rader (SAR) instruments identify oil-covered waters by measuring the suppressed radio waves caused by the dampened capillary waves (Krestenitis, Marios, et al, 2019). However, there is a problem of incorrect classification of lookalike areas (water surfaces with low reflectance rates): oil slicks are extremely diverse in shape and size. Correcting false positives requires further processing, such as multi-segmentation schemes and semi-supervised detection methods. This wastes valuable time, and leaves potential for efforts being directed to the wrong area. By combining SAR images with deep convolutional neural networks (DCNN), researchers hope to establish highly accurate, automatic classifications of oil slick areas, false positive lookalikes, and unaffected sea surfaces.
Deep Convolutional Neural Networks
Deep Convolutional Neural Networks (DCNNs) work as fully connected feedforward models which can be trained to learn the relationship between satellite observations and target values. They work as a regression problem, automatically constructing the complex relationship from input to output in a hierarchical manner from an encoded compressed feature vector to a segmented result that matches the dimensions of the original image. Complex features can then be quickly extracted from initially identified regions, reducing overlap between true and false positives (2019).
Methods & Materials
In the study, SAR images from European Space Agency (ESA) and Copernicus Open Access hub were used as a common base of comparison and confirmed areas of oil spills were obtained from European Maritime Safety Agency to be used as ‘ground truth’ data (EMSA). 1112 Sentinel-1 C-band images were split into 90% training and 10% testing to evaluate five DCNN methods (U-Net, LinkNet, PSPNet, DeepLabv2, DeepLabv3+) across five classifications (land, water, lookalikes, oil spill, ships). DeepLabv3 displayed the best overall performance: testing accuracy was improved to 89.0% from an initial value of 79.7% (2019). DeepLabv3’s processing time was also kept low due to its lower GPU allocation requirements.
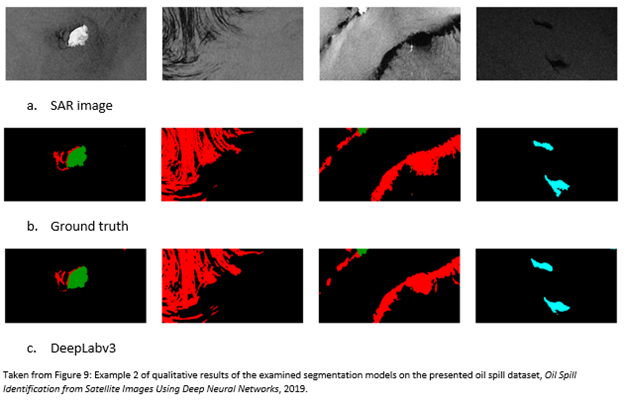
Similar results have been reported from other studies: a 2018 paper using side-looking airbourne radar (SLAR) images and a two-stage convolutional neural network obtained a 99% accuracy, while a 2020 study using SAR images and the OSCNet DCNN produced a 94% accuracy rating (Shabab etal, 2021). These studies cited similar challenges with target evaluation due to the dynamic nature of oil slicks caused by variable wind speeds and sea currents, yet their results assert the importance of including additional convolutional neural network methods.
Rival Remote Sensing Method
Another method of oil spill identification that has gained attention is the use of airbourne-mounted laser fluorosensors. They work by identifying the absorption and emission wavelengths unique to oil (Fingas & Brown, 2017). They are especially useful for discriminating between oiled and unoiled seaweeds and shorelines and are often considered an essential part of a remote sensing package (2017). However, airbourne missions can be expensive and may not be suitable in more dangerous weather settings. Furthermore, they are a sampling tool: their resolution is controlled by the laser repetition rate and the speed of the aircraft. Sentinel-1, however, has a repeat time of six days and can penetrate cloud cover.
Conclusions
The primary response objectives are to prevent spill from reaching shore, reduce impact on marine life, and speed degradation of unrecovered oil (Oil Spill Prevention and Response, 2021). The responsible agents use booms to block and direct oil on the surface, chemical dispersants to degrade oil and in some cases burn off excess oil. Effective cleanup requires timely information on the shape and size of the oil slick and proximity to shorelines and fragile ecosystems. The combination of SAR imagery with DCNN technology provides decision makers with a fast, accurate, trustworthy, and relatively easily repeatable mechanism for identifying and evaluating oil spills on the ocean surface. Initial responses are enhanced, costs are abated, and future cleanup can be significantly reduced: all stakeholders benefit from increased investment in rapid oil spill detection using advanced remote sensing techniques like DCNN.
Works Cited
“2020 Accomplishments: The Year in Review.” Response.restoration.noaa.gov, National Oceanic and Atmospheric Administration, 10 Sept. 2021, response.restoration.noaa.gov/2020-accomplishments.
“Clean Up: Oceans.” Oil Spill Prevention & Response, 2021, www.oilspillprevention.org/oil-spill-cleanup/ocean-oil-spill-cleanup.
Cohen, Mark A. A Taxonomy of Oil Spill Costs. Resources for the Future, June 2010, citeseerx.ist.psu.edu/viewdoc/summary?doi=10.1.1.622.5418.
David M. Uhlmann & Jeffrey F. Liss. “BP Paid a Steep Price for the Gulf Oil Spill but for the Us a Decade Later, It’s Business as Usual.” The Conversation, 5 Jan. 2021, theconversation.com/bp-paid-a-steep-price-for-the-gulf-oil-spill-but-for-the-us-a-decade-later-its-business-as-usual-136905.
Fingas, Merv, and Carl Brown. “A Review of Oil Spill Remote Sensing.” Sensors, vol. 18, no. 2, ser. 91, 30 Sept. 2017. 91, doi:10.3390/s18010091.
Krestenitis, Marios, et al. “Oil Spill Identification from Satellite Images Using Deep Neural Networks.” Remote Sensing, vol. 11, no. 15, 26 June 2019, doi:10.3390/rs11151762.
Shaban, Mohamed, et al. “A Deep-Learning Framework for the Detection of Oil Spills From Sar Data.” Sensors, vol. 21, no. 7, 28 Mar. 2021, p. 2351., doi:10.3390/s21072351.
Varghese, Arpan, et al. “U.S. Probing Nearly 350 Reports of Oil Spills in Wake of Hurricane Ida.” Reuters, Thomson Reuters, 7 Sept. 2021, www.reuters.com/world/us/us-probing-nearly-350-reports-oil-spills-wake-hurricane-ida-2021-09-06/.
There’s a quite a lot to learn from you and your blogs, awesome sharing thank you so much.