Walkability and Deep learning
Walkability is about how friendly the neighbourhood is to walk. Walkability holds many advantages in terms of environment, health, and economy. Walking was the primary mode of transportation spanning human civilization when cars were not widely accessible and relied upon. The streets of the cities were designed keeping in mind the pedestrians and their interconnectivity with the community’s commercial core for easy ingress to services and commodities. Huge amounts of effort have gone into understanding and institutionalizing the design of transportation space for motorized vehicles during the last 60 years. Pedestrian transportation, on the other hand, is a much newer addition to urban planning procedures, and it is currently treated with considerably less vigour, seriousness, and financing (Lo, 2009).
Some new initiatives as shown in figure1 have been brought forward for urban streets like the complete streets approach by smart growth America, the SWAT (the Scottish Walkability Assessment Tool) platform in the UK, and the green streets approach in Portland (Li, 2020). With Growing advances, a focus on walkability in human-centred street spaces is among the most important environmental remedial actions for modern metropolitan areas.
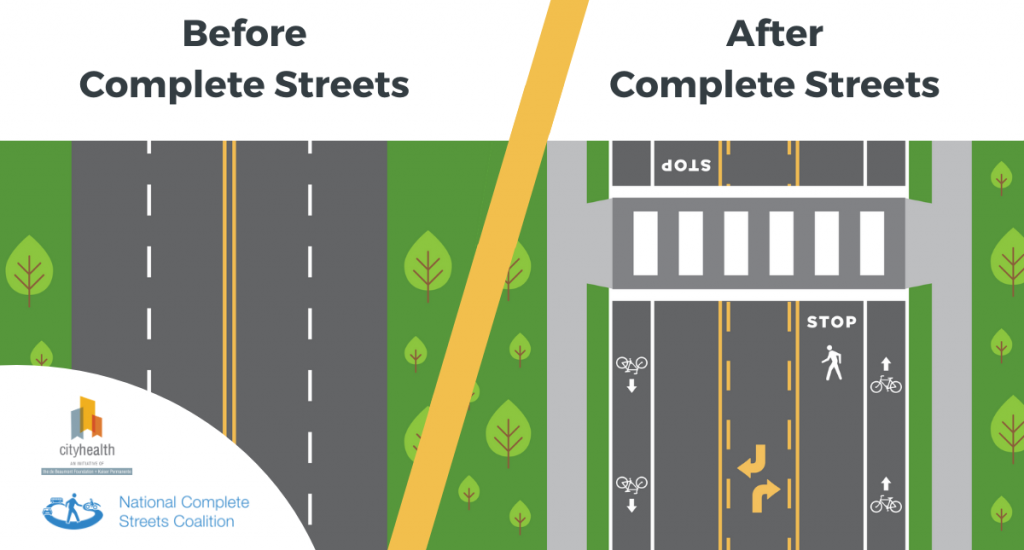
Figure 1: Before and after Complete Street (Complete Streets, n.d.)
The Walk score also known as the walkability index rates the walkability of a location by assessing hundreds of walking routes to local facilities and giving points depending on the route to services in every category. The highest number of scores are awarded to facilities and services within a 5-minute walk (0.25 mile). The farther distant the facilities are, the fewer points they earn; services that are more than 1.5 miles (30-minute walk) away receive no points (How Walk Score Works, n.d.). Numbers between 0 to 100 are considered Walk scores. As per the general guidelines of interpreting walk score, the numbers between 90-100 are walker’s paradise, 70-89 is very walkable, 50-69 is somewhat walkable, 25-49 is car-dependent, and 0-24 is Car-dependent for driving only. In recent times, the publicly accessible website walk score, https://www.walkscore.com/, was found to be legitimate and useful for evaluating access to neighbouring walkable facilities (Carr et al., 2010). It showed the use of walk score as a simple, straightforward, and rapid substitute for local density and amenities. An example has been given to measure the walk score of the University of Ottawa in figure 2.
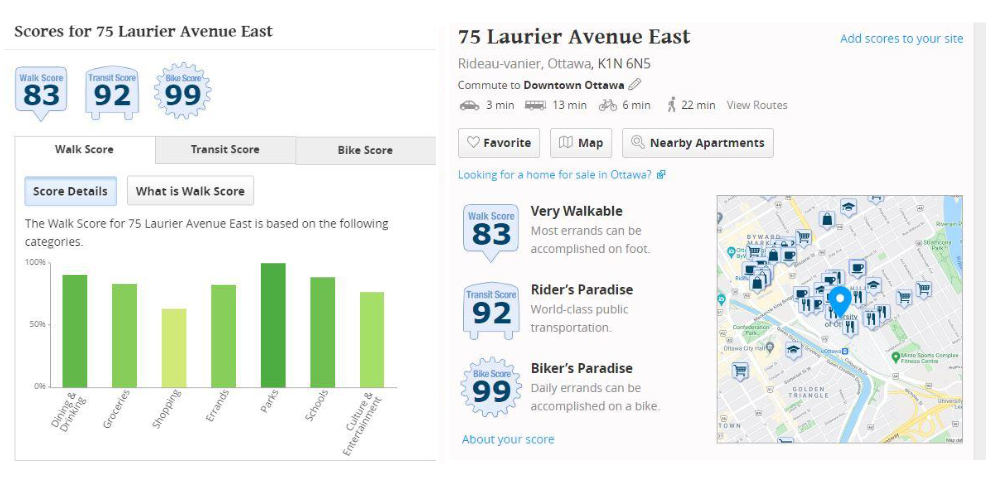
Figure 2: University of Ottawa’s walk score based on different categories (Source: (How Walk Score Works, n.d.))
Deep learning is a subset of machine learning in which artificial neural networks are trained on massive volumes of data to discover patterns and make choices. It has been used successfully for a variety of applications like object recognition, natural language processing, and predictive analysis (What Is Deep Learning and How Does It Work? n.d.). Deep learning can be utilized in the field of walkability to evaluate and analyze huge quantities of information mostly on the built environment, commuter facilities, and land use patterns in order to forecast walkability scores for various places. This can be accomplished by providing data to the neural network on parameters such as the quality and availability of pathways and sidewalks, the accessibility of places and facilities, and the volume and speed of traffic on surrounding roadways. This information can then be used by the neural network to learn correlations and patterns between these characteristics and walkability, as well as generate predictions about the walkability of different locations.
The study of researchers of the University of Maryland, who constructed a deep neural model that predicted walkability ratings for areas in Baltimore using the information on the physical surroundings and urban infrastructure, is a such illustration of the utilization of deep learning for pedestrian evaluation (Casagrande, n.d.). The model predicted walkability scores with an accuracy of more than 90%, indicating the ability of deep learning to effectively assess walkability.
Deep learning can be used to recognize trends and patterns in walkability with time, as well as factors that have contributed to shifts in walkability, in addition to forecasting walkability scores. This can be valuable for lawmakers and developers who are aiming to increase walkability in their community since it can aid them in comprehending what elements are more than important to take into account when making adjustments to the urban landscape or land use trends.
To conclude, deep learning does have the ability to change the way we analyze and promote walkability in our cities. Deep learning can assist us in better comprehending the factors that lead to walkability and making more educated decisions by evaluating enormous volumes of data on building design, commuter facilities, and land use patterns.
References:
Carr, L. J., Dunsiger, S. I., & Marcus, B. H. (2010). Walk ScoreTM As a Global Estimate of Neighborhood Walkability. American Journal of Preventive Medicine, 39(5), 460–463.
https://doi.org/10.1016/j.amepre.2010.07.007
Casagrande, S. S. (n.d.). WALKABILITY, HEALTHY FOOD AVAILABILITY AND THE ASSOCIATION WITH OBESITY AND DIABETES IN BALTIMORE CITY, MARYLAND.
Complete Streets. (n.d.). Smart Growth America. Retrieved May 29, 2022, from https://smartgrowthamerica.org/what-are-complete-streets/
How Walk Score Works. (n.d.). Retrieved November 7, 2021, from https://www.walkscore.com/live-more/canada/
Li, Y. (2020). A big data evaluation of urban street walkability using deep learning and environmental sensors—A case study around Osaka University Suita campus. Werner, L and Koering, D (Eds.), Anthropologic: Architecture and Fabrication in the Cognitive Age – Proceedings of the 38th ECAADe Conference – Volume 2, TU Berlin, Berlin, Germany, 16-18 September 2020, Pp. 319-328. http://papers.cumincad.org/cgi-bin/works/paper/ecaade2020_113
Lo, R. H. (2009). Walkability: What is it? Journal of Urbanism: International Research on
Placemaking and Urban Sustainability, 2(2), 145–166.
https://doi.org/10.1080/17549170903092867
What is Deep Learning and How Does It Work? (n.d.). Enterprise AI. Retrieved December 19, 2022, from https://www.techtarget.com/searchenterpriseai/definition/deep-learning-deep-neural-network